New Survey: Broad Expert Consensus for Many AGI Safety and Governance Practices
We recently surveyed 51 leading experts from AGI labs, academia, and civil society. We found overwhelming support for many AGI safety and governance practices, including pre-deployment risk assessments, dangerous capabilities evaluations, third-party model audits, safety restrictions on model usage, and red teaming.
Jonas Schuett, Noemi Dreksler, Markus Anderljung, David McCaffary, Lennart Heim, Emma Bluemke, Ben Garfinkel
This post summarises our recent paper “Towards Best Practices in AGI Safety and Governance.” You can read the full paper and see the complete survey results here.
GovAI research blog posts represent the views of their authors, rather than the views of the organisation.
Summary
- We found a broad consensus that AGI labs should implement most of the safety and governance practices in a 50-point list. For every practice but one, the majority of respondents somewhat or strongly agreed that it should be implemented. Furthermore, for the average practice on our list, 85.2% somewhat or strongly agreed it should be implemented.
- Respondents agreed especially strongly that AGI labs should conduct pre-deployment risk assessments, dangerous capabilities evaluations, third-party model audits, safety restrictions on model usage, and red teaming. 98% of respondents somewhat or strongly agreed that these practices should be implemented. On a numerical scale, ranging from -2 to 2, each of these practices received a mean agreement score of at least 1.76.
- Experts from AGI labs had higher average agreement with statements than respondents from academia or civil society. However, no significant item-level differences were found.
Significant risks require comprehensive best practices
Over the past few months, a number of powerful and broadly capable artificial intelligence (AI) systems have been released and integrated into products used by millions of people, including search engines and major digital work suites like Google Workspace or Microsoft 365. As a result, policymakers and the public have taken an increasing interest in emerging risks from AI.
These risks are likely to grow over time. A number of leading companies developing these AI systems, including OpenAI, Google DeepMind, and Anthropic1, have the stated goal of building artificial general intelligence (AGI)—AI systems that achieve or exceed human performance across a wide range of cognitive tasks. In pursuing this goal, they may develop and deploy AI systems that pose particularly significant risks. While they have already taken some measures to mitigate these risks, best practices have not yet emerged.
A new survey of AGI safety and governance experts
To support the identification of best practices, we sent a survey to 92 leading experts from AGI labs, academia, and civil society and received 51 responses (55.4% response rate). You can find the names of the 33 experts that gave permission to be listed publicly as participants of the survey below, along with the full statements and more information about the sample and methodology.
Participants were asked how much they agreed with 50 statements about what AGI labs should do on a Likert scale: “Strongly agree”, “somewhat agree”, “neither agree nor disagree”, “somewhat disagree”, “strongly disagree”, and “I don’t know”. We explained to the participants that, by “AGI labs”, we primarily mean organisations that have the stated goal of building AGI. This includes OpenAI, Google DeepMind, and Anthropic. Since other AI companies like Microsoft and Meta conduct similar research (e.g. training very large and general-purpose models), we also classify them as “AGI labs” in the survey and this post.
You can see our key results in the figure below.

Broad consensus for a large portfolio of practices
There was a broad consensus that AGI labs should implement most of the safety and governance practices in the 50-point list. For 98% of the practices, a majority (more than 50%) of respondents strongly or somewhat agreed. For 56% of the practices, a majority (more than 50%) of respondents strongly agreed. The mean agreement across all 50 items was 1.39 on a scale from -2 (strongly disagree) to 2 (strongly agree)—roughly halfway between somewhat agree and strongly agree. On average, across all 50 items, 85.2% of respondents either somewhat or strongly agreed that AGI labs should follow each of the practices.
On average, only 4.6% either somewhat or strongly disagreed that AGI labs should follow each of the practices. For none of the practices, a majority (more than 50%) of respondents somewhat or strongly disagreed. Indeed, the highest total disagreement on any item was 16.2% for the item “avoid capabilities jumps”. Across all 2,285 ratings respondents made, only 4.5% were disagreement ratings.
Pre-deployment risk assessments, dangerous capabilities evaluations, third-party model audits, safety restrictions, and red teaming have overwhelming expert support
The statements with the highest mean agreement were: pre-deployment risk assessment (mean = 1.9), dangerous capabilities assessments (mean = 1.9), third-party model audits (mean = 1.8), safety restrictions (mean = 1.8), and red teaming (mean = 1.8). The items with the highest total agreement proportions all had agreement ratings from 98% of respondents were: dangerous capabilities evaluations, internal review before publication, monitor systems and their uses, pre-deployment risk assessment, red teaming, safety restrictions, and third-party model audits. Seven items had no disagreement ratings at all: dangerous capabilities evaluations, industry sharing of security information, KYC screening, pre-deployment risk assessment, publish alignment strategy, safety restrictions, and safety vs. capabilities.
The figure below shows the statements with the highest and lowest mean agreement. Note that all practices, even those with lowest mean agreement, show a positive mean agreement, that is above the midpoint of “neither agree nor disagree” and in the overall agreement part of the scale. The mean agreement for all statements can be seen in Figure 3 in the Appendix.

Experts from AGI labs had higher average agreement for practices
Overall, it appears that experts closest to the technology show the highest average agreement with the practices. We looked at whether respondents differed in their responses by sector (AGI labs, academia, civil society) and gender (man, woman). Respondents from AGI labs (mean = 1.54) showed significantly higher mean agreement than respondents from academia (mean = 1.16) and civil society (mean = 1.36).
There was no significant difference in overall mean agreement between academia and civil society. We found no significant differences between sector groups for any of the items. We also found no significant differences between responses from men and women—neither in overall mean agreement, nor at the item level. Please see the full paper for details on the statistical analyses conducted.
Research implications
In light of the broad agreement on the practices presented, future work needs to figure out the details of these practices. There is ample work to be done in determining the practical execution of these practices and how to make them a reality. Respondents also suggested 50 more practices, highlighting the wealth of AGI safety and governance approaches that need to be considered beyond the ones asked about in this survey. This work will require a collaborative effort from both technical and governance experts.
Policy implications
The findings of the survey have a variety of implications for AGI labs, regulators, and standard-setting bodies:
- AGI labs can use our findings to conduct an internal gap analysis to identify potential best practices that they have not yet implemented. For example, our findings can be seen as an encouragement to make or follow through on commitments to commission third-party model audits, evaluate models for dangerous capabilities, and improve their risk management practices.
- In the US, where the US government has recently expressed concerns about the dangers of AI and AGI, regulators and legislators can use our findings to prioritise different policy interventions. In the EU, our findings can inform the debate on to what extent the proposed AI Act should account for general-purpose AI systems. In the UK, our findings can be used to draft upcoming AI regulations as announced in the recent white paper “A pro-innovation approach to AI regulation” and to put the right guardrails in place for frontier AI systems.
- Our findings can inform an ongoing initiative of the Partnership on AI to develop shared protocols for the safety of large-scale AI models. They can also support efforts to adapt the NIST AI Risk Management Framework and ISO/IEC 23894 to developers of general-purpose AI systems. Finally, they can inform the work of CEN-CENELEC—a cooperation between two of the three European Standardisation Organisations— to develop harmonised standards for the proposed EU AI Act, especially on risk management.
- Since most practices are not inherently about AGI labs, our findings might also be relevant for other organisations that develop and deploy increasingly general-purpose AI systems, even if they do not have the goal of building AGI.
Conclusion
Our study has elicited current expert opinions on safety and governance practices at AGI labs, providing a better understanding of what leading experts from AGI labs, academia, and civil society believe these labs should do to reduce risk. We have shown that there is broad consensus that AGI labs should implement most of the 50 safety and governance practices we asked about in the survey. For example, 98% of respondents somewhat or strongly agreed that AGI labs should conduct pre-deployment risk assessments, evaluate models for dangerous capabilities, commission third-party model audits, establish safety restrictions on model usage, and commission external red teams. Ultimately, our list of practices may serve as a helpful foundation for efforts to develop best practices, standards, and regulations for AGI labs.
Recently, US Vice President Kamala Harris invited the chief executive officers of OpenAI, Google DeepMind, Anthropic, and other leading AI companies to the White House “to share concerns about the risks associated with AI”. In an almost 3-hour long Senate hearing on May 16th 2023, Sam Altman, the CEO of OpenAI, was asked to testify on the risks of AI and the regulations needed to mitigate these risks. We believe that now is a pivotal time for AGI safety and governance. Experts from many different domains and intellectual communities must come together to discuss what responsible AGI labs should do.
Citation
Schuett, J., Dreksler, N., Anderljung, M., McCaffary, D., Heim, L., Bluemke, E., & Garfinkel, B. (2023, 5th June). New survey: Broad expert consensus for many AGI safety and governance practices. Centre for the Governance of AI. www.governance.ai/post/broad-expert-consensus-for-many-agi-safety-and-governance-best-practices.
If you have questions or would like more information regarding the policy implications of this work, please contact jonas.schuett@governance.ai. To find out more about the Centre for the Governance of AI’s survey research, please contact noemi.dreksler@governance.ai.
Acknowledgements
We would like to thank all participants who filled out the survey. We are grateful for the research assistance and in-depth feedback provided by Leonie Koessler and valuable suggestions from Akash Wasil, Jeffrey Laddish, Joshua Clymer, Aryan Bhatt, Michael Aird, Guive Assadi, Georg Arndt, Shaun Ee, and Patrick Levermore. All remaining errors are our own.
Appendix
List of statements
Below, we list all statements we used in the survey, sorted by overall mean agreement. Optional statements, that respondents could choose to answer or not, are marked with an asterisk (*).
- Pre-deployment risk assessment. AGI labs should take extensive measures to identify, analyze, and evaluate risks from powerful models before deploying them.
- Dangerous capability evaluations. AGI labs should run evaluations to assess their models' dangerous capabilities (e.g. misuse potential, ability to manipulate, and power-seeking behavior).
- Third-party model audits. AGI labs should commission third-party model audits before deploying powerful models.
- Safety restrictions. AGI labs should establish appropriate safety restrictions for powerful models after deployment (e.g. restrictions on who can use the model, how they can use the model, and whether the model can access the internet).
- Red teaming. AGI labs should commission external red teams before deploying powerful models.
- Monitor systems and their uses. AGI labs should closely monitor deployed systems, including how they are used and what impact they have on society.
- Alignment techniques. AGI labs should implement state-of-the-art safety and alignment techniques.
- Security incident response plan. AGI labs should have a plan for how they respond to security incidents (e.g. cyberattacks).*
- Post-deployment evaluations. AGI labs should continually evaluate models for dangerous capabilities after deployment, taking into account new information about the model’s capabilities and how it is being used.*
- Report safety incidents. AGI labs should report accidents and near misses to appropriate state actors and other AGI labs (e.g. via an AI incident database).
- Safety vs capabilities. A significant fraction of employees of AGI labs should work on enhancing model safety and alignment rather than capabilities.
- Internal review before publication. Before publishing research, AGI labs should conduct an internal review to assess potential harms.
- Pre-training risk assessment. AGI labs should conduct a risk assessment before training powerful models.
- Emergency response plan. AGI labs should have and practice implementing an emergency response plan. This might include switching off systems, overriding their outputs, or restricting access.
- Protection against espionage. AGI labs should take adequate measures to tackle the risk of state-sponsored or industrial espionage.*
- Pausing training of dangerous models. AGI labs should pause the development process if sufficiently dangerous capabilities are detected.
- Increasing level of external scrutiny. AGI labs should increase the level of external scrutiny in proportion to the capabilities of their models.
- Publish alignment strategy. AGI labs should publish their strategies for ensuring that their systems are safe and aligned.*
- Bug bounty programs. AGI labs should have bug bounty programs, i.e. recognize and compensate people for reporting unknown vulnerabilities and dangerous capabilities.
- Industry sharing of security information. AGI labs should share threat intelligence and information about security incidents with each other.*
- Security standards. AGI labs should comply with information security standards (e.g. ISO/IEC 27001 or NIST Cybersecurity Framework). These standards need to be tailored to an AGI context.
- Publish results of internal risk assessments. AGI labs should publish the results or summaries of internal risk assessments, unless this would unduly reveal proprietary information or itself produce significant risk. This should include a justification of why the lab is willing to accept remaining risks.*2
- Dual control. Critical decisions in model development and deployment should be made by at least two people (e.g. promotion to production, changes to training datasets, or modifications to production).*
- Publish results of external scrutiny. AGI labs should publish the results or summaries of external scrutiny efforts, unless this would unduly reveal proprietary information or itself produce significant risk.*
- Military-grade information security. The information security of AGI labs should be proportional to the capabilities of their models, eventually matching or exceeding that of intelligence agencies (e.g. sufficient to defend against nation states).
- Board risk committee. AGI labs should have a board risk committee, i.e. a permanent committee within the board of directors which oversees the lab’s risk management practices.*
- Chief risk officer. AGI labs should have a chief risk officer (CRO), i.e. a senior executive who is responsible for risk management.
- Statement about governance structure. AGI labs should make public statements about how they make high-stakes decisions regarding model development and deployment.*
- Publish views about AGI risk. AGI labs should make public statements about their views on the risks and benefits from AGI, including the level of risk they are willing to take in its development.
- KYC screening. AGI labs should conduct know-your-customer (KYC) screenings before giving people the ability to use powerful models.*
- Third-party governance audits. AGI labs should commission third-party audits of their governance structures.*
- Background checks. AGI labs should perform rigorous background checks before hiring/appointing members of the board of directors, senior executives, and key employees.*
- Model containment. AGI labs should contain models with sufficiently dangerous capabilities (e.g. via boxing or air-gapping).
- Staged deployment. AGI labs should deploy powerful models in stages. They should start with a small number of applications and fewer users, gradually scaling up as confidence in the model’s safety increases.
- Tracking model weights. AGI labs should have a system that is intended to track all copies of the weights of powerful models.*
- Internal audit. AGI labs should have an internal audit team, i.e. a team which assesses the effectiveness of the lab’s risk management practices. This team must be organizationally independent from senior management and report directly to the board of directors.
- No open-sourcing. AGI labs should not open-source powerful models, unless they can demonstrate that it is sufficiently safe to do so.3
- Researcher model access. AGI labs should give independent researchers API access to deployed models.
- API access to powerful models. AGI labs should strongly consider only deploying powerful models via an application programming interface (API).
- Avoiding hype. AGI labs should avoid releasing powerful models in a way that is likely to create hype around AGI (e.g. by overstating results or announcing them in attention-grabbing ways).
- Gradual scaling. AGI labs should only gradually increase the amount of compute used for their largest training runs.
- Treat updates similarly to new models. AGI labs should treat significant updates to a deployed model (e.g. additional fine-tuning) similarly to its initial development and deployment. In particular, they should repeat the pre-deployment risk assessment.
- Pre-registration of large training runs. AGI labs should register upcoming training runs above a certain size with an appropriate state actor.
- Enterprise risk management. AGI labs should implement an enterprise risk management (ERM) framework (e.g. the NIST AI Risk Management Framework or ISO 31000). This framework should be tailored to an AGI context and primarily focus on the lab's impact on society.
- Treat internal deployments similarly to external deployments. AGI labs should treat internal deployments (e.g. using models for writing code) similarly to external deployments. In particular, they should perform a pre-deployment risk assessment.*4
- Notify a state actor before deployment. AGI labs should notify appropriate state actors before deploying powerful models.
- Notify affected parties. AGI labs should notify parties who will be negatively affected by a powerful model before deploying it.*
- Inter-lab scrutiny. AGI labs should allow researchers from other labs to scrutinize powerful models before deployment.*
- Avoid capabilities jumps. AGI labs should not deploy models that are much more capable than any existing models.*
- Notify other labs. AGI labs should notify other labs before deploying powerful models.*
List of Participants
- Allan Dafoe, Google DeepMind
- Andrew Trask, University of Oxford, OpenMined
- Anthony M. Barrett
- Brian Christian, Author and Researcher at UC Berkeley and University of Oxford
- Carl Shulman, Advisor, Open Philanthropy
- Chris Meserole, Brookings Institution
- Gillian Hadfield, University of Toronto, Schwartz Reisman Institute for Technology and Society
- Hannah Rose Kirk, University of Oxford
- Holden Karnofsky, Open Philanthropy
- Iason Gabriel, Google DeepMind
- Irene Solaiman, Hugging Face
- James Bradbury, Google DeepMind
- James Ginns, Centre for Long-Term Resilience
- Jason Clinton, Anthropic
- Jason Matheny, RAND
- Jess Whittlestone, Centre for Long-Term Resilience
- Jessica Newman, UC Berkeley AI Security Initiative
- Joslyn Barnhart, Google DeepMind
- Lewis Ho, Google DeepMind
- Luke Muehlhauser, Open Philanthropy
- Mary Phuong, Google DeepMind
- Noah Feldman, Harvard University
- Robert Trager, Centre for the Governance of AI
- Rohin Shah, Google DeepMind
- Sean O hEigeartaigh, Centre for the Future of Intelligence, University of Cambridge
- Seb Krier, Google DeepMind
- Shahar Avin, Centre for the Study of Existential Risk, University of Cambridge
- Stuart Russell, UC Berkeley
- Tantum Collins
- Toby Ord, University of Oxford
- Toby Shevlane, Google DeepMind
- Victoria Krakovna, Google DeepMind
- Zachary Kenton, Google DeepMind
Additional figures
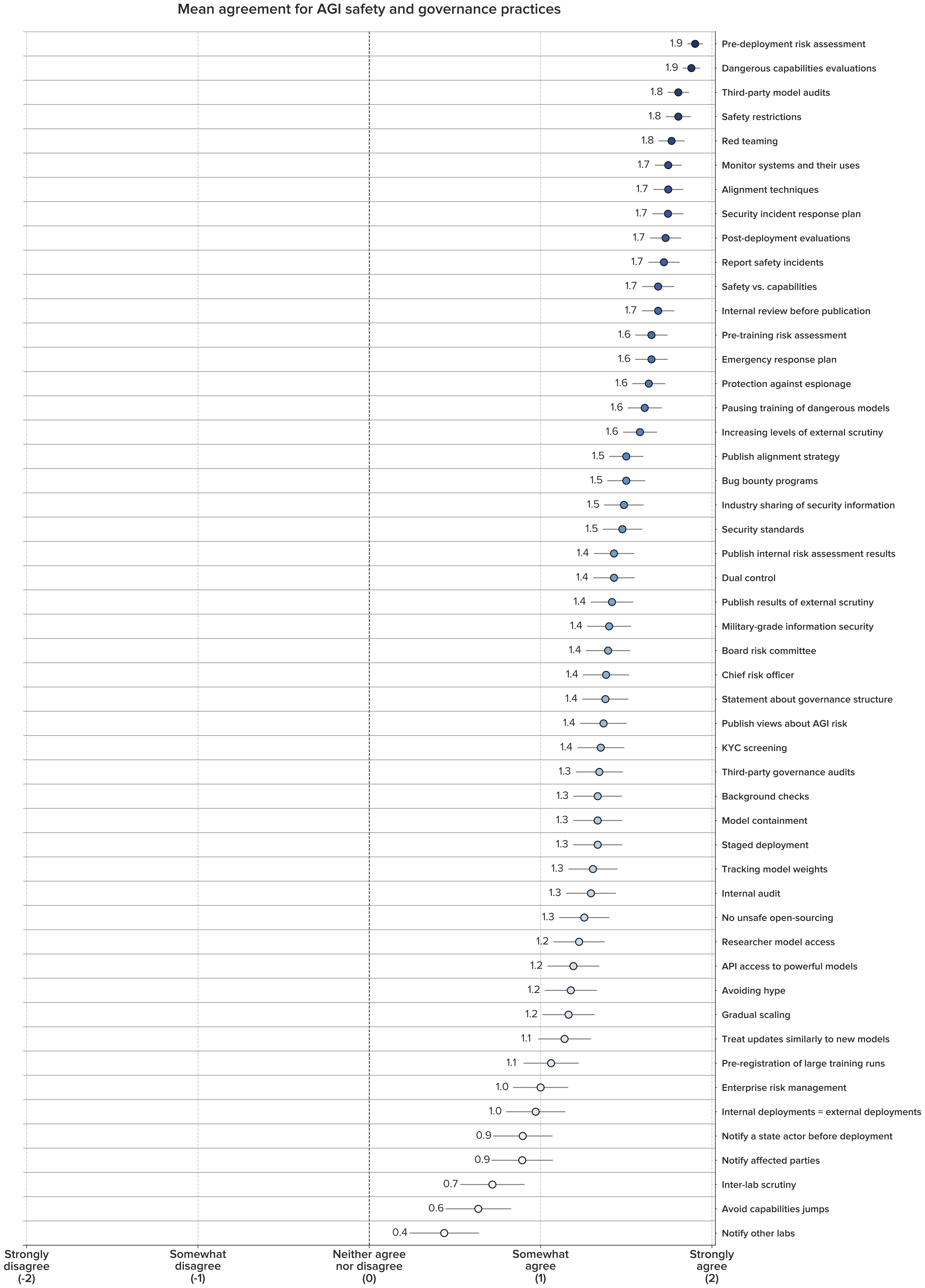
Methodology
Open science
The survey draft, pre-registration, pre-analysis plan, code, and data can be found on OSF. To protect the identity of respondents, we will not make any demographic data or text responses public. We largely followed the pre-analysis plan. The full methodology, along with any deviations from the pre-registered analyses, can be found in the full paper.
Sample
Our sample could best be described as a purposive sample. We selected individual experts based on their knowledge and experience in areas relevant for AGI safety and governance, but we also considered their availability and willingness to participate. We used a number of proxies for expertise, such as the number, quality, and relevance of their publications as well as their role at relevant organisations. The breakdown of the sample by sector and gender can be seen in Figure 4. Overall, we believe the selection reflects an authoritative sample of current AGI safety and governance-specific expertise. However, there are a variety of limitations of the sample detailed in our full paper.
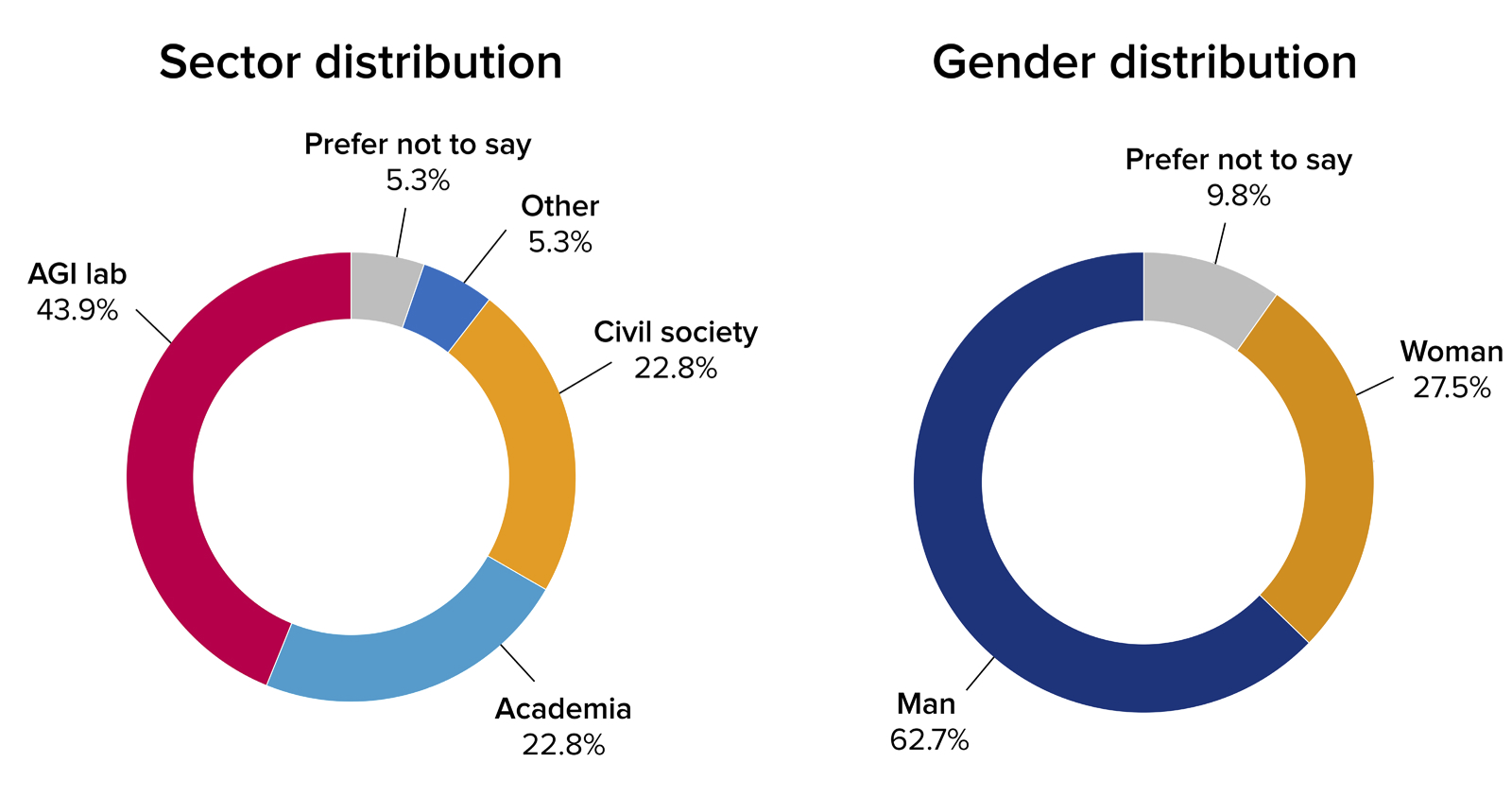
Survey distribution
The survey took place between 26 April and 8 May 2023. Informed consent had to be given before proceeding to the main survey. Responses to the survey were anonymous.
Footnotes
1 Anthropic does not use the term “AGI” in their public communication. They mainly talk about “frontier models” or “transformative AI systems” (Anthropic, 2023). However, Anthropic is generally considered to pursue the goal of building AGI.
2 Labelled as “Publish internal risk assessment results” in some figures due to space constraints.
3 Throughout this post and the paper, we changed the title of this item to “no unsafe open-sourcing” to avoid misconceptions.
4 Labelled as “Internal deployments = external deployments” in some figures due to space constraints.